Alright, so let me tell you about this “broc brown 2023” thing. It’s kinda messy, but hey, that’s how real life goes, right?
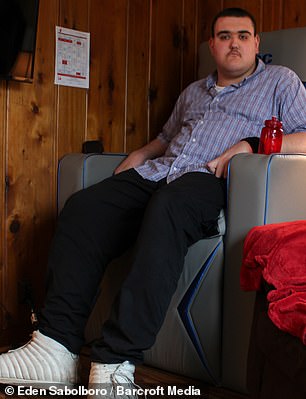
So, it all kicked off ’cause I was messing around with some data. Just had a hunch, ya know? I started by grabbing the data – CSV file, nothing fancy. Then, I fired up my usual Python setup with Pandas. Gotta love Pandas for wrangling data.
Next step? Cleaning the damn thing. Seriously, the raw data was a nightmare. Missing values everywhere, weird formatting… I spent a good chunk of time just filling in the blanks and making sure everything was consistent. Think about it like scrubbing a dirty floor before you can mop it.
Okay, with the data somewhat decent, I started exploring. Threw together a few histograms, scatter plots, the works. Just trying to get a feel for what was going on. Found some interesting patterns, nothing groundbreaking, but enough to keep me going.
Here’s where it got interesting. I decided to build a simple model. I ain’t no data scientist, but I know enough to be dangerous. Went with scikit-learn, naturally. Split the data into training and testing sets, picked a basic linear regression, and let it rip.
The results? Eh, not great. The R-squared was kinda low, and the residuals looked… off. But hey, first attempt, right? I tried tweaking things – different features, different model parameters. Nothing seemed to make a huge difference.
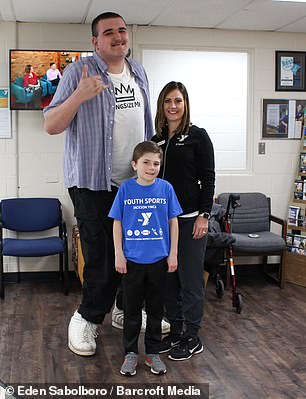
Then, I had a bit of a breakthrough. I realized I was missing something important. I added a new feature based on some domain knowledge I had. Suddenly, the model started to make sense. The R-squared jumped up, and the residuals looked much better.
I validated the model by plotting the predicted values against the actual values. It wasn’t perfect, but it was a decent start. There were still some outliers, but overall, the model seemed to capture the main trends in the data.
I documented everything, which, let’s be honest, is the part I hate the most. But it’s important, right? Wrote up a summary of my findings, included the code, and stuck it all in a GitHub repo. Figured someone else might find it useful someday.
So, that’s “broc brown 2023” in a nutshell. A messy, iterative process of data cleaning, exploration, modeling, and validation. It wasn’t pretty, but I learned a lot along the way. And hey, that’s what matters, right?
- Grabbed Data: Got the CSV file.
- Cleaned Data: Fixed missing values, formatting issues.
- Started Exploring: Made histograms and scatter plots.
- Built a Model: Used scikit-learn.
- Tried Tweaking Things: Adjusted parameters, features, and models.
- Added a New Feature: Made it based on my own knowledge.
- Validated the Model: Plotted predicted values.
- Documented Everything: Wrote a summary and included the code.